Convolutional U-Net models can be trimmed and tuned to
fit on miniature computers, enabling real-time inference within medical devices.
Introduction#
In spring 2023, I took CS231n: Deep Learning for Computer Vision at Stanford. My group of three chose to explore volumetric (“3-D”) segmentation of MRI images. Using 2020 data from the University of Pennsylvania’s Brain Tumor Segmentation (BraTS) Challenge, we trained and experimented with multiple U-Net architectures.
The dataset consisted of 369 labelled examples each containing 5 MRIs:
- Four input scans: T2 FLAIR, T1, T1 contrast enhanced, T2
- One mask of four classes:
non-tumor (
0b000
), non-enhancing tumor core (0b001
), peritumoral edema (0b010
), and Gadolinium-enhancing tumor (0b100
)
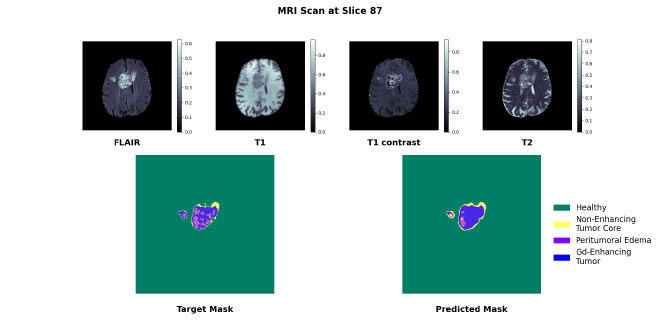
U-Net Architecture#
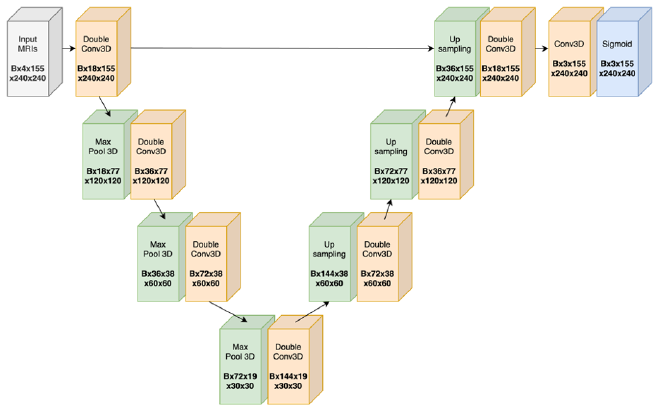
One can choose to use 3-D or 2-D convolutions in the U-Net:
- 3-D convolution: can directly intake a 3-D MRI and leverage 3-D spatial information, at the cost of 3X more weights
- 2-D convolution: an MRI now becomes a list of 2-D images, so to process an MRI the model is internally doing a nested for loop
We experimented with both 2-D and 3-D convolutions.
One note is, because the output of the sigmoid is logits, the loss function used was the equally-weighted sum of binary cross entropy (with logits) loss and Dice loss.
Binary Threshold Tuning#
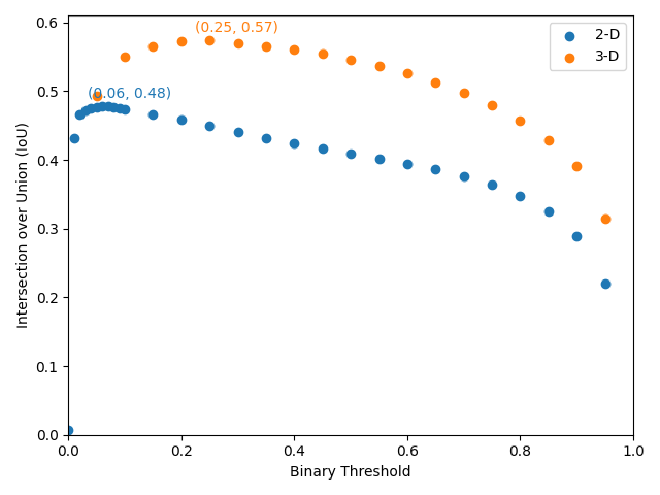
Other Findings#

- 3-D convolutional layers outperform 2-D layers, indicating 3-D U-Nets are actually leveraging 3-D spatial information
- Applying one global binary threshold to convert raw predictions to class predictions is equally performant as per-mask binary thresholding
- Global parameter pruning,
encoder-decoder pair pruning,
and reducing weight precision (
float32
tofloat16
) were effective methods of reducing model size without hampering performance
Source Code#
jamesbraza/cs231n-3d-segmentation
Stanford CS231N Deep Learning for Computer Vision Class Project
Python
0
0